Unlocking Success with Advanced Data Annotation Tools & Platforms for Machine Learning
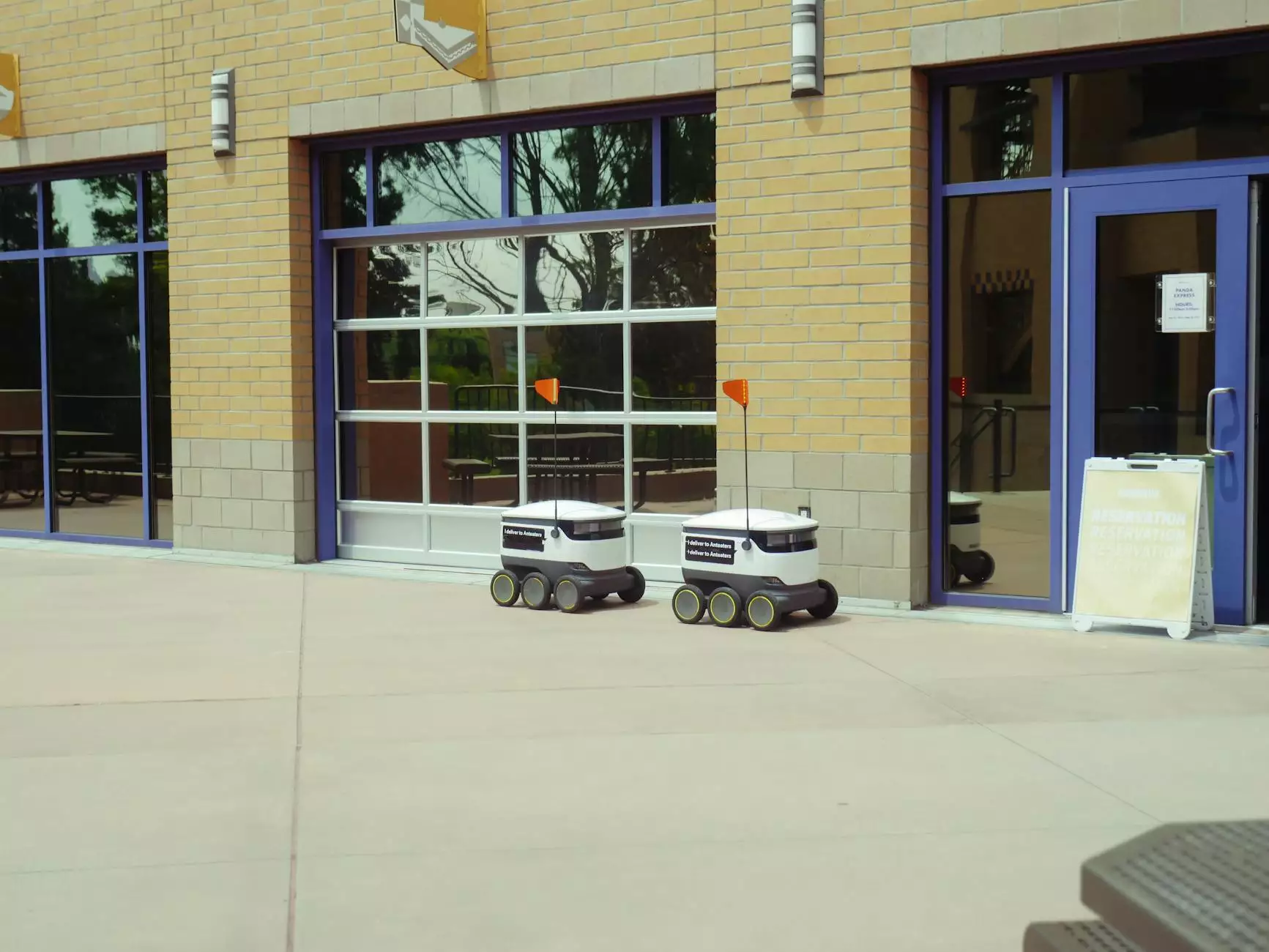
In the rapidly evolving realm of artificial intelligence (AI) and machine learning (ML), the foundation of highly accurate models lies in the quality and quantity of data available for training. Among various data types, images play a pivotal role in applications like autonomous vehicles, medical diagnostics, security systems, and many other innovative fields. To harness the full potential of image data, businesses rely on sophisticated data annotation tools and platforms that enable precise labeling, categorization, and structuring of visual information. One such innovative solution is keylabs.ai, leading the way with comprehensive data annotation platform offerings tailored for today’s AI needs.
Why Data Annotation is Critical for Machine Learning Success
Data annotation refers to the process of labeling raw data — in this case, images — to make it understandable by machine learning algorithms. Imagine training an autonomous vehicle to recognize pedestrians; without properly annotated images to distinguish humans from other objects, the vehicle's perception system would be unreliable. Effective annotation ensures that models can learn from real-world characteristics, crave accuracy, and perform robustly in diverse scenarios.
Key Benefits of Proper Data Annotation for Businesses
- Enhanced Model Accuracy: Well-annotated data enables models to recognize patterns more precisely, reducing errors and improving predictive capabilities.
- Accelerated Development Cycle: Automated annotation tools and platforms reduce manual effort, allowing faster iteration and deployment of AI solutions.
- Cost Efficiency: Reducing label noise and minimizing the need for rework translates into lower overall development costs.
- Regulatory Compliance: Accurate annotations are crucial for adhering to industry standards and legal requirements, especially in healthcare and security domains.
- Competitive Advantage: High-quality data annotation directly correlates with superior model performance, giving businesses an edge in the market.
The Evolution of Data Annotation Tools and Platforms
From manual labeling to highly automated platforms, the evolution of data annotation tools reflects the increasing complexity and volume of data-driven projects. Early approaches involved labor-intensive tasks performed by skilled annotators, often leading to bottlenecks and inconsistencies. Today, advanced platforms like keylabs.ai leverage artificial intelligence, machine learning-assisted annotation, and collaborative workflows to optimize data labeling processes.
Core Features of Leading Data Annotation Platforms
- Intuitive User Interface: Simplifies complex annotation tasks for both experts and non-technical annotators.
- Support for Multiple Data Types: Covering images, videos, text, and 3D data to cater to diverse AI applications.
- Annotation Automation: AI-assisted suggestions and semi-automatic labeling to expedite workflows.
- Collaborative Tools: Enable teams to work seamlessly across geographies with version control and task management features.
- Quality Control Mechanisms: Built-in validation tools to ensure high annotation accuracy and consistency.
- Integration Capabilities: Compatibility with popular ML frameworks, data storage, and cloud services for streamlined pipelines.
Specialization in Image Annotation for Machine Learning
Among various data modalities, image annotation for machine learning stands out as a cornerstone for training visual perception models. Precise image labels help AI systems accurately identify objects, boundaries, textures, and contextual relationships. This process involves several types of annotations, including bounding boxes, polygons, semantic segmentation, keypoints, and more, depending on the application's complexity.
Types of Image Annotation Techniques
- Bounding Boxes: Draw rectangles around objects of interest, suitable for object detection tasks like identifying cars, pedestrians, or animals.
- Polygonal Annotation: Outline complex shapes with detailed polygons, essential for accurate segmentation of irregular objects.
- Semantic Segmentation: Assign a label to every pixel in an image, enabling pixel-perfect understanding for tasks like medical imaging.
- Keypoint Annotation: Mark specific points on objects, such as joints in human pose estimation or facial landmarks.
- Instance Segmentation: Combine object detection and segmentation to distinguish individual object instances within a scene.
Why Choose a Specialized Data Annotation Platform Like keylabs.ai?
Implementing image annotation for machine learning at scale requires a platform that balances accuracy, speed, and ease of use. keylabs.ai is designed specifically to meet these needs, offering solutions that empower AI teams to produce high-quality labeled datasets efficiently.
Key Advantages of keylabs.ai
- Advanced Automation: AI-assisted annotation reduces manual effort and accelerates project timelines.
- High-Quality Standards: Rigorous validation processes and expert oversight ensure data accuracy.
- Flexible Workflow Management: Customizable pipelines adapt to project requirements, whether small-scale or enterprise-wide.
- Global Collaboration: Multilingual interfaces and distributed teams facilitate seamless teamwork across borders.
- Cost-Effectiveness: Scalable solutions optimize resource usage, minimizing overhead costs.
The Future of Data Annotation in Machine Learning
The landscape of data annotation is continuously evolving with technological advances. Emerging trends include:
- AI-Driven Self-Labeling: Systems that learn from minimal human input to auto-generate annotations with high confidence.
- Active Learning: Intelligent algorithms that identify the most informative samples for annotation, reducing total labeling effort.
- Integrated Annotation and Model Training: End-to-end platforms that streamline the data-to-model pipeline for faster deployment.
- Quality Assurance Enhancements: Real-time validation tools that detect and correct inconsistent labels.
- Augmented Reality (AR) & Virtual Reality (VR) Annotation: Extending annotation capabilities into 3D and immersive environments, opening new avenues for AI training.
How Businesses Can Maximize ROI from Data Annotation Platforms
Effective utilization of data annotation platforms like keylabs.ai requires strategic planning. Here are factors to consider:
- Clearly Define Project Goals: Determine the specific model objectives and annotation requirements upfront.
- Invest in Quality Control: Implement validation and review workflows to maintain high data accuracy.
- Leverage Automation: Use AI-assisted tools to handle repetitive tasks and focus human effort on complex labeling.
- Build a Skilled Annotation Team: Train staff for consistency and familiarity with annotation standards.
- Iterate and Improve: Regularly analyze model performance to identify gaps in data quality and adjust annotation strategies accordingly.
Conclusion: Elevate Your AI Projects with Superior Data Annotation
In conclusion, the success of any machine learning initiative heavily depends on the quality of annotated data, especially for complex visual tasks involving images. With advanced data annotation tools and platforms like keylabs.ai, organizations can unlock powerful AI capabilities by ensuring data accuracy, efficiency, and scalability. Embracing innovation in data annotation not only accelerates development cycles but also provides a competitive edge in the AI-driven economy.
Investing in top-tier image annotation for machine learning solutions is a strategic move that directly translates into smarter models, better insights, and ultimately, greater business success in the digital age.